WP6- In silico clinical trials
Integration and validation
Watch this video by Nerea Arrarte, Sara Bridio and Claire Miller (April 2022) on In silico Clinical trials for the treatment of acute ischaemic stroke
This WP will integrate the virtual population models from WP2 with the thrombolysis modelling from WP3, the thrombectomy modelling from WP4, and the perfusion and brain tissue damage modelling from WP5 into the INSIST in silico clinical trials for acute ischemic stroke. To achieve this goal, we will set up a suitable computing environment that seamlessly carries out in silico clinical trials. We will perform, in close collaboration with WP2-WP5, a formal Verification, Validation, and Uncertainty Quantification of the fully integrated in silico clinical trial, and finally run three in silico clinical trials, addressing the three R&D questions described below;
Trial initiator | Hypothesis | Study design |
Medical device industry | Finer smaller grained configurations of stent-retrievers reduce thrombus fragmentation and thus result in an improvement of treatment of patients with acute ischemic stroke | In silico randomized clinical trial |
Pharma industry | Does the reduction of active Thrombin-Activatable Fibrinolysis Inhibitor (TAFIa) improve recanalization of tissue plasminogen activator or improve microvascular reperfusion after thrombectomy? | In silico randomized clinical trial |
Treating health professionals | Patients with a high-risk of large vessel occlusion stroke benefit from an early start of thrombectomy before the administration of thrombolysis. | In silico randomized clinical trial |
Description of three clinically relevant hypotheses to be addressed by in silico clinical trials
Objectives:
1. Integrate the in silico models into an in silico clinical trial for acute ischemic stroke.
2. Validate using formal VVUQ procedures;
3. Apply the resulting in silico clinical trial to three relevant R&D questions.
Update September 2020
Current work in WP6 continues the implementation and improvement of the framework, and determining methodologies for the VVUQ procedures. Figure 1 shows an overview of the data flow between the different models (and WPs) for the trial.
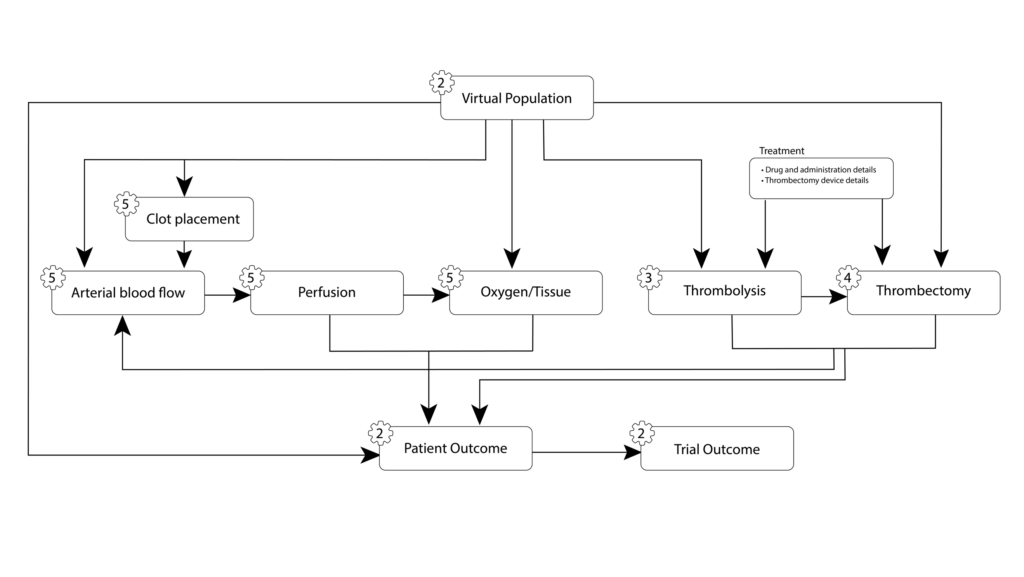
Figure 1. The trial models and the arrows show the data transfer between them.
The INSIST framework uses containers (Docker or Singularity) for each of the models in order to maximise portability between computer environments and assist with reproducibility. A command line interface has been developed for the easy creation and running of trials, with patient specifications detailed in a patient YAML file. Simple parallel support has also been added to enable running larger trial cohorts.
Preliminary uncertainty quantification (UQ) activities have begun for the VVUQ of the trial. In order to easily implement the UQ methodologies, the EasyVVUQ framework, developed within the VECMA project, was integrated into the INSIST trial framework. We then undertook preliminary UQ analysis of the blood flow model in both baseline and occluded state, using a Quasi-Monte Carlo approach. Four parameters were considered: blood viscosity, density, stroke volume, and heart rate. Give the uncertainties in the inputs shown in Figure 2 (the histograms show the sample points used for the analysis), we can determine the sensitivity of the output of interest, in this case flow rate in the occluded vessel, to each of these uncertainties. This is shown in Figure 3. Note however, these results are preliminary and shown to demonstrate the use of EasyVVUQ with the INSIST framework.
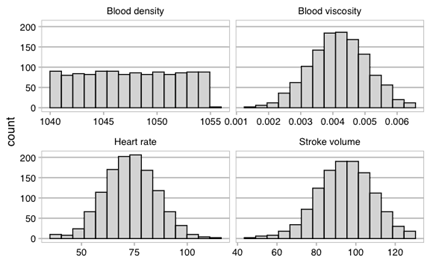
Figure 2. Histograms of the sample points for the uncertainties in the inputs.
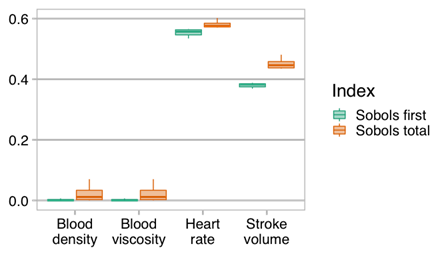
Figure 3. Sobols first order and total effect indices showing the significance of the uncertainties in each of the parameters investigated in the uncertainty quantification. Higher values indicate a higher sensitivity to the input.